Forecasting transformative AI: the "biological anchors" method in a nutshell
This is one of 4 posts summarizing hundreds of pages of technical reports focused almost entirely on forecasting one number: the year by which transformative AI will be developed.1By "transformative AI," I mean "AI powerful enough to bring us into a new, qualitatively different future." I specifically focus on what I'm calling PASTA: AI systems that can essentially automate all of the human activities needed to speed up scientific and technological advancement.
The sooner PASTA might be developed, the sooner the world could change radically, and the more important it seems to be thinking today about how to make that change go well vs. poorly.
This post is a layperson-compatible summary of Ajeya Cotra's "Forecasting Transformative AI with Biological Anchors" (which I'll abbreviate below as "Bio Anchors"), and its pros and cons.2 It is the forecast I find most informative for transformative AI, with some caveats:
- This approach is relatively complex, and it requires a fairly large number of assumptions and uncertain estimates. These qualities make it relatively difficult to explain, and they are also a mark against the method's reliability.
- Hence, as of today, I don't think this method is as trustworthy as the examples I gave previously for forecasting a qualitatively different future. It does not have the simplicity and directness of some of those examples, such as modeling COVID-19's spread. And while climate modeling is also very complex, climate modeling has been worked on by a large number of experts over decades, whereas the Bio Anchors methodology doesn't have much history.
Nonetheless, I think it is the best available "best guess estimate" methodology for transformative AI timelines as of today. And as discussed in the final section, one can step back from a lot of the details to see that this century will likely see us hit some of the more "extreme" milestones in the report that strongly suggest the feasibility of transformative AI.
(Note: I've also written up a follow-up post about this framework for skeptical readers. See “Biological anchors” is about bounding, not pinpointing, AI timelines.)
The basic idea is:
- Modern AI models can "learn" to do tasks via a (financially costly) process known as "training." You can think of training as a massive amount of trial-and-error. For example, voice recognition AI models are given an audio file of someone talking, take a guess at what the person is saying, then are given the right answer. By doing this millions of times, they "learn" to reliably translate speech to text. More: Training
- The bigger an AI model and the more complex the task, the more the training process costs. Some AI models are bigger than others; to date, none are anywhere near "as big as the human brain" (what this means will be elaborated below). More: Model size and task type
- The biological anchors method asks: "Based on the usual patterns in how much training costs, how much would it cost to train an AI model as big as a human brain to perform the hardest tasks humans do? And when will this be cheap enough that we can expect someone to do it?" More: Estimating the expense
Bio Anchors models a broad variety of different ways of approaching this question, generating estimates in a wide range from "aggressive" (projecting transformative AI sooner) to "conservative" (later). But from essentially all of these angles, it places a high probability on transformative AI this century.
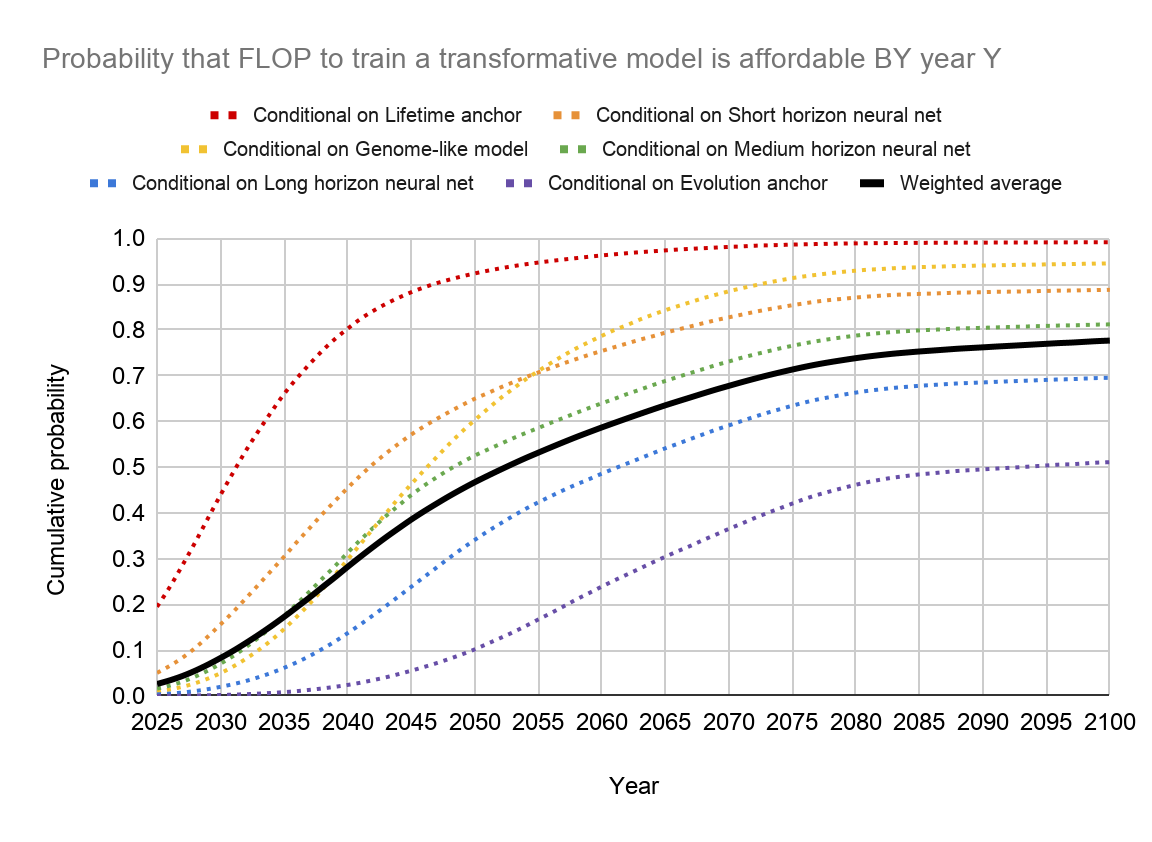
I'll now elaborate on each of these a bit more. This is the densest part of this series, and some people might prefer to stick with the above summary and skip to the next post.
Note that Bio Anchors uses a number of different approaches (which it calls "anchors") to estimate transformative AI timelines, and combines them into one aggregate view. In this summary, I'm most focused on a particular set of these - called the "neural net anchors" - which are driving most of the report's aggregate timelines. Some of what I say applies to all anchors, but some applies only to the "neural net anchors."
Training
As discussed previously, there are essentially two ways to "teach" a computer to do a task:
- "Program" in extremely specific, step-by-step instructions for completing the task. When this can be done, the computer can generally execute the instructions very quickly, reliably and cheaply. For example, you might program a computer to examine each record in a database and print the ones that match a user's search terms - you would "instruct" it in exactly how to do this, and it would be able to do the task very well.
- "Train" an AI to do the task purely by trial and error. Today, the most common way of doing this is by using a "neural network," which you might think of sort of like a "digital brain" that starts in a random state: it hasn't yet been wired to do specific things. For example, say we want an AI to be able to say whether a photo is of a dog or a cat. It's hard to give fully specific step-by-step instructions for doing this; instead, we can take a neural network and send in a million example images (each one labeled as a "dog" or a "cat"). Each time it sees an example, it will tweak its internal wiring to make it more likely to get the right answer on similar cases in the future. After enough examples, it will be wired to correctly recognize dogs vs. cats.
(We could maybe also move up another level of meta, and try to "train" models to be able to learn from "training" itself as efficiently as possible. This is called "meta-learning," but my understanding is that it hasn't had great success yet.)
"Training" is a sort of brute-force, expensive alternative to "programming." The advantage is that we don't need to be able to provide specific instructions - we can just give an AI lots of examples of doing the task right, and it will learn to do the task. The disadvantage is that we need a lot of examples, which requires a lot of processing power, which costs money.
How much? This depends on the size of the model (neural network) and the nature of the task itself. For some tasks AIs have learned as of 2021, training a single model could cost millions of dollars. For more complex tasks (such as "do innovative scientific research") and bigger models (reaching the size of the human brain), training a model could cost far more than that.
Bio Anchors is interested in the question: "When will it be affordable to train a model, using a relatively crude trial-and-error-based approach, to do the hardest tasks humans can do?"
These tasks could include the tasks necessary for PASTA, such as:
- Learn about science from teachers, textbooks and homework as effectively as a human can.
- Push the frontier of science by asking questions, doing analyses and writing papers, as effectively as a human can.
The next section will discuss how Bio Anchors fleshes out the idea of the "hardest tasks humans can do" (which it assumes would require a "human-brain-sized" model).
Model size and task type
Bio Anchors hypothesizes that we can estimate "how expensive it is to train a model" based on two basic parameters: the model size and the task type.
Model size. As stated above, you might think of a neural network as a "digital brain" that starts in a random state. In general, a larger "digital brain" - with more digital-versions-of-neurons and digital-versions-of-synapses3 - can learn more complex tasks. A larger "digital brain" also requires more computations - and is hence more expensive - each time it is used (for example, for each example it is learning from).
Drawing on the analysis in Joe Carlsmith's "How Much Computational Power Does It Take to Match the Human Brain?" (abbreviated in this piece as "Brain Computation"), Bio Anchors estimates comparisons between the size of "digital brains" (AI models) and "animal brains" (bee brains, mouse brains, human brains). These estimates imply that today's AI systems are sometimes as big as insect brains, but never quite as big as mouse brains - as of this writing, the largest known language model was the first to come reasonably close4 - and not yet even 1% as big as human brains.5
The bigger the model, the more processing power it takes to train. Bio Anchors assumes that a transformative AI model would need to be about 10x the size of a human brain, so a lot bigger than any current AI model. (The 10x is to leave some space for the idea that "digital brains" might be less efficient than human brains; see this section of the report.) This is one of the reasons it would be very expensive to train.
It could turn out that a smaller AI model is still big enough to learn the above sort of tasks. Or it could turn out that the needed model size is bigger than Bio Anchors estimates, perhaps because Bio Anchors has underestimated the effective "size" of the human brain, or because the human brain is better-designed than "digital brains" by more than Bio Anchors has guessed.
Task type. In order to learn a task, an AI model needs to effectively "try" (or "watch") the task a large number of times, learning from trial-and-error. The more costly (in processing power, and therefore money) the task is to try/watch, the more costly it will be for the AI model to learn it.
It's hard to quantify how costly a task is to try/watch. Bio Anchors's attempt to do this is the most contentious part of the analysis, according to the technical reviewers who have reviewed it so far.
You can roughly think of the Bio Anchors framework as saying:
- There are some tasks that a human can do with only a second of thought, such as classifying an image as a cat or dog.
- There are other tasks that might take a human several minutes of thought, such as solving a logic puzzle.
- Other tasks could take hours, days, months or even years, and require not just thinking, but interacting with the environment. For example, writing a scientific paper.
- The tasks on the longer end of this spectrum will be more costly to try/watch, so it will be more costly to train an AI model to do them. For example, it's more costly (takes more time, and more money) to have a million "tries" at a task that takes an hour than it is to have a million "tries" at a task that takes a second.
- However, the framework isn't as simple as this sounds. Many tasks that seem like "long" tasks (such as writing an essay) could in fact be broken into a series of "shorter" tasks (such as writing individual sentences).
- If an AI model can be trained to do a shorter "sub-task,", it might be able to do the longer task by simply repeating the shorter sub-task over and over again - without ever needing to be explicitly "trained" to do the longer task.
- For example, an AI model might get a million "tries" at the task: "Read a partly-finished essay and write a good next sentence." If it then learns to do this task well, it could potentially write a long essay by simply repeating this task over and over again. It wouldn't need to go into a separate training process where it gets a million "tries" at the more time-consuming task of writing an entire essay.
- So it becomes crucial whether the hardest and most important tasks (such as those listed above) are the kind that can be "decomposed" into short/easy tasks.
Estimating the expense
Bio Anchors looks at how expensive existing AI models were to train, depending on model size and task type (as defined above). It then extrapolates this to see how expensive an AI model would be to train if it:
- Had a size 10x larger than a human brain.6
- Trained on a task where each "try" took days, weeks, or months of intensive "thinking."
As of today, this sort of training would cost in the ballpark of a million trillion dollars, which is enormously more than total world wealth. So it isn't surprising that nobody has tried to train such a model.
However, Bio Anchors also projects the following trends out into the future:
- Advances in both hardware and software that could make computing power cheaper.
- A growing economy, and a growing role of AI in the economy, that could increase the amount AI labs are able to spend training large models to $1 trillion and beyond.
According to these projections, at some point the "amount AI labs are able to spend" becomes equal to the "expense of training a human-brain-sized model on the hardest tasks." Bio Anchors bases its projections for "when transformative AI will be developed" on when this happens.
Bio Anchors also models uncertainty in all of the parameters above, and considers alternative approaches to the "model size and task type" parameters.7 By doing this, it estimates the probability that transformative AI will be developed by 2030, 2035, etc.
Aggressive or conservative?
Bio Anchors involves a number of simplifications that could cause it to be too aggressive (expecting transformative AI to come sooner than is realistic) or too conservative (expecting it to come later than is realistic).
The argument I most commonly hear that it is "too aggressive" is along the lines of: "There's no reason to think that a modern-methods-based AI can learn everything a human does, using trial-and-error training - no matter how big the model is and how much training it does. Human brains can reason in unique ways, unmatched and unmatchable by any AI unless we come up with fundamentally new approaches to AI." This kind of argument is often accompanied by saying that AI systems don't "truly understand" what they're reasoning about, and/or that they are merely imitating human reasoning through pattern recognition.
I think this may turn out to be correct, but I wouldn't bet on it. A full discussion of why is outside the scope of this post, but in brief:
- I am unconvinced that there is a deep or stable distinction between "pattern recognition" and "true understanding" (this Slate Star Codex piece makes this point). "True understanding" might just be what really good pattern recognition looks like. Part of my thinking here is an intuition that even when people (including myself) superficially appear to "understand" something, their reasoning often (I'd even say usually) breaks down when considering an unfamiliar context. In other words, I think what we think of as "true understanding" is more of an ideal than a reality.
- I feel underwhelmed with the track record of those who have made this sort of argument - I don't feel they have been able to pinpoint what "true reasoning" looks like, such that they could make robust predictions about what would prove difficult for AI systems. (For example, see this discussion of Gary Marcus's latest critique of GPT3, and similar discussion on Astral Codex Ten).
- "Some breakthroughs / fundamental advances are needed" might be true. But for Bio Anchors to be overly aggressive, it isn't enough that some breakthroughs are needed; the breakthroughs needed have to be more than what AI scientists are capable of in the coming decades, the time frame over which Bio Anchors forecasts transformative AI. It seems hard to be confident that things will play out this way - especially because:
- Even moderate advances in AI systems could bring more talent and funding into the field (as is already happening8).
- If money, talent and processing power are plentiful, and progress toward PASTA is primarily held up by some particular weakness of how AI systems are designed and trained, a sustained attempt by researchers to fix this weakness could work. When we're talking about multi-decade timelines, that might be plenty of time for researchers to find whatever is missing from today's techniques.
More broadly, Bio Anchors could be too aggressive due to its assumption that "computing power is the bottleneck":
- It assumes that if one could pay for all the computing power to do the brute-force "training" described above for the key tasks (e.g., automating scientific work), transformative AI would (likely) follow.
- Training an AI model doesn't just require purchasing computing power. It requires hiring researchers, running experiments, and perhaps most importantly, finding a way to set up the "trial and error" process so that the AI can get a huge number of "tries" at the key task. It may turn out that doing so is prohibitively difficult.
On the other hand, there are several ways in which Bio Anchors could be too conservative (underestimating the likelihood of transformative AI being developed soon).
- Perhaps with enough ingenuity, one could create a transformative AI by "programming" it to do key tasks, rather than having to "train" it (see above for the distinction). This could require far less computation, and hence be far less expense. Or one could use a combination of "programming" and "training" to achieve better efficiency than Bio Anchors implies, while still not needing to capture everything via "programming."
- Or one could find far superior approaches to AI that can be "trained" much more efficiently. One possibility here is "meta-learning": effectively training an AI system on the "task" of being trained, itself.
- Or perhaps most likely, over time AI might become a bigger and bigger part of the economy, and there could be a proliferation of different AI systems that have each been customized and invested in to do different real-world tasks. The more this happens, the more opportunity there is for individual ingenuity and luck to result in more innovations, and more capable AI systems in particular economic contexts.
- Perhaps at some point, it will be possible to integrate many systems with different abilities in order to tackle some particularly difficult task like "automating science," without needing a dedicated astronomically expensive "training run."
- Or perhaps AI that falls short of PASTA will still be useful enough to generate a lot of cash, and/or help researchers make compute cheaper and more efficient. This in turn could lead to still bigger AI models that further increase availability of cash and efficiency of compute. That, in turn, could cause a PASTA-level training run to be affordable earlier than Bio Anchors projects.
- Additionally, some technical reviewers of Bio Anchors feel that its treatment of task type is too conservative. They believe that the most important tasks (and perhaps all tasks) that AI needs to be trained on will be on the "easier/cheaper" end of the spectrum, compared to what Bio Anchors assumes. (See the above section for what it means for a task to be "easier/cheaper" or "harder/more expensive"). For a related argument, see Fun with +12 OOMs of Compute, which makes the intuitive point that Bio Anchors is imagining a truly massive amount of computation needed to create PASTA, and less could easily be enough.
I don't think it is obvious whether, overall, Bio Anchors is too aggressive (expecting transformative AI to come sooner than is realistic) or too conservative (expecting it to come later). The report itself states that it's likely to be too aggressive over the next few years and too conservative >50 years out, and likely most useful in between.9
Intellectually, it feels to me as though the report is more likely to be too conservative. I find its responses to the "Too aggressive" points above fairly compelling, and I think the "Too conservative" points are more likely to end up being correct. In particular, I think it's hard to rule out the possibility of ingenuity leading to transformative AI in some far more efficient way than the "brute-force" method contemplated here. And I think the treatment of "task type" is definitely erring in a conservative direction.
However, I also have an intuitive preference (which is related to the "burden of proof" analyses given previously) to err on the conservative side when making estimates like this. Overall, my best guesses about transformative AI timelines are similar to those of Bio Anchors.
Conclusions of Bio Anchors
Bio Anchors estimates a >10% chance of transformative AI by 2036, a ~50% chance by 2055, and an ~80% chance by 2100.
It's also worth noting what the report says about AI systems today. It estimates that:
- Today's largest AI models, such as GPT-3, are a bit smaller than mouse brains, and are starting to get within range (if they were to grow another 100x-1000x) of human brains. So we might soon be getting close to AI systems that can be trained to do anything that humans can do with ~1 second of thought. Consistent with this, it seems to me that we're just starting to reach the point where language models sound like humans who are talking without thinking very hard.10 If anything, "human who puts in no more than 1 second of thought per word" seems somewhat close to what GPT-3 is doing, even though it's much smaller than a human brain.
- It's only very recently that AI models have gotten this big. A "large" AI model before 2020 would be more in the range of a honeybee brain. So for models even in the very recent past, we should be asking whether AI systems seem to be "as smart as insects." Here's one attempt to compare AI and honeybee capabilities (by Open Philanthropy intern Guille Costa), concluding that the most impressive honeybee capabilities the author was able to pinpoint do appear to be doable for AI systems.11
I include these notes because:
- The Bio Anchors analysis seems fully consistent with what we're observing from AI systems today (and have over the last decade or two), while also implying that we're likely to see more transformative abilities in the coming decades.
- I think it's particularly noteworthy that we're getting close to the time when an AI model is "as big as a human brain" (according to the Bio Anchors / Brain Computation estimation method). It may turn out that such an AI model is able to "learn" a lot about the world and produce a lot of economic value, even if it can't yet do the hardest things humans do. And this, in turn, could kick off skyrocketing investment in AI (both money and talent), leading to a lot more innovation and further breakthroughs. This is a simple reason to believe that transformative AI by 2036 is plausible.
Finally, I note that Bio Anchors includes an "evolution" analysis among the different approaches it considers. This analysis hypothesizes that in order to produce transformative AI, one would need to do about as many computations as all animals in history combined, in order to re-create the progress that was made by natural selection.
I consider the "evolution" analysis to be very conservative, because machine learning is capable of much faster progress than the sort of trial-and-error associated with natural selection. Even if one believes in something along the lines of "Human brains reason in unique ways, unmatched and unmatchable by a modern-day AI," it seems that whatever is unique about human brains should be re-discoverable if one is able to essentially re-run the whole history of natural selection. And even this very conservative analysis estimates a ~50% chance of transformative AI by 2100.
Pros and cons of the biological anchors method for forecasting transformative AI timelines
Cons. I'll start with what I see as the biggest downside: this is a very complex forecasting framework, which relies crucially on multiple extremely uncertain estimates and assumptions, particularly:
- Whether it's reasonable to believe that an AI system could learn the key tasks listed above (the ones required for PASTA) given enough trial-and-error training.
- How to compare the size of AI models with the size of animal/human brains.
- How to characterize "task type," estimating how "difficult" and expensive a task is to “try” or “watch” once.
- How to use the model size and task type to estimate how expensive it would be to train an AI model to do the key tasks.
- How to estimate future advances in both hardware and software that could make computing power cheaper.
- How to estimate future increases in how much AI labs could be able to spend training models.
This kind of complexity and uncertainty means (IMO) that we shouldn't consider the forecasts to be highly reliable, especially today when the whole framework is fairly new. If we got to the point where as much scrutiny and effort had gone into AI forecasting as climate forecasting, it might be a different matter.
Pros. That said, the biological anchors method is essentially the only one I know of that estimates transformative AI timelines from objective facts (where possible) and explicit assumptions (elsewhere).12 It does not rely on any concepts as vague and intuitive as "how fast AI systems are getting more impressive" (discussed previously). Every assumption and estimate in the framework can be explained, discussed, and - over time - tested.
Even in its current early stage, I consider this a valuable property of the biological anchors framework. It means that the framework can give us timelines estimates that aren't simply rehashes of intuitions about whether it feels as though transformative AI is approaching.13
I also think it's encouraging that even with all the guesswork, the testable "predictions" the framework makes as of today seem reasonable (see previous section). The framework provides a way of thinking about how it could be simultaneously true that (a) the AI systems of a decade ago didn't seem very impressive at all; (b) the AI systems of today can do many impressive things but still feel far short of what humans are able to do; (c) the next few decades - or even the next 15 years - could easily see the development of transformative AI.
Additionally, I think it's worth noting a couple of high-level points from Bio Anchors that don't depend on quite so many estimates and assumptions:
- In the coming decade or so, we're likely to see - for the first time - AI models with comparable "size" to the human brain.
- If AI models continue to become larger and more efficient at the rates that Bio Anchors estimates, it will probably become affordable this century to hit some pretty extreme milestones - the "high end" of what Bio Anchors thinks might be necessary. These are hard to summarize, but see the "long horizon neural net" and "evolution anchor" frameworks in the report.
- One way of thinking about this is that the next century will likely see us go from "not enough compute to run a human-sized model at all" to "extremely plentiful compute, as much as even quite conservative estimates of what we might need." Compute isn't the only factor in AI progress, but to the extent other factors (algorithms, training processes) became the new bottlenecks, there will likely be powerful incentives (and multiple decades) to resolve them.
A final advantage of Bio Anchors is that we can continue to watch AI progress over time, and compare what we see to the report's framework. For example, we can watch for:
- Whether there are some tasks that just can't be learned, even with plenty of trial and error - or whether some tasks require amounts of training very different from what the report estimates.
- How AI models' capabilities compare to those of animals that we are currently modeling as "similarly sized." If AI models seem more capable than such animals, we may be overestimating how large a model we would need to be in order to e.g. automate science. If they seem less capable, we may be underestimating it.
- How hardware and software are progressing, and whether AI models are getting bigger at the rate the report currently projects.
The next piece will summarize all of the different analyses so far about transformative AI timelines. It will then discuss a remaining reservation: that there is no robust expert consensus on this topic.
Next in series: AI Timelines: Where the Arguments, and the "Experts," Stand
Use "Feedback" if you have comments/suggestions you want me to see, or if you're up for giving some quick feedback about this post (which I greatly appreciate!) Use "Forum" if you want to discuss this post publicly on the Effective Altruism Forum.
Footnotes
-
Of course, the answer could be "A kajillion years from now" or "Never." ↩
-
For transparency, note that this is an Open Philanthropy analysis, and I am co-CEO of Open Philanthropy. ↩
-
I (like Bio Anchors) generally consider the synapse count more important than the neuron count, for reasons I won't go into here. ↩
-
Wikipedia: "GPT-3's full version has a capacity of 175 billion machine learning parameters ... Before the release of GPT-3, the largest language model was Microsoft's Turing NLG, introduced in February 2020, with a capacity of 17 billion parameters." Wikipedia doesn't state this, but I don't believe there are publicly known AI models larger than these language models (with the exception of "mixture-of-experts models" that I think we should disregard for these purposes, for reasons I won't go into here). Wikipedia estimates about 1 trillion synapses for a house mouse's brain; Bio Anchors's methodology for brain comparisons (based on Brain Computation) essentially equates synapses to parameters. ↩
-
Bio Anchors estimates about 100 trillion parameters for the human brain, based on the fact that it has about 100 trillion synapses. ↩
-
As noted above, the 10x is to leave some space for the idea that "digital brains" might be less efficient than human brains. See this section of the report. ↩
-
For example, one approach hypothesizes that training could be made cheaper by "meta-learning," discussed above; another approach hypothesizes that in order to produce transformative AI, one would need to do about as many computations as all animals in history combined, in order to re-create the progress that was made by natural selection.) ↩
-
See charts from the early sections of the 2021 AI Index Report, for example. ↩
-
See this section. ↩
-
In fact, he estimates that AI systems appear to use about 1000x less compute, which would match the above point in terms of suggesting that AI systems might be more efficient than animal/human brains and that the Bio Anchors estimates might be too conservative. However, he doesn't address the fact that bees arguably perform a more diverse set of tasks than the AI systems they're being compared to. ↩
-
Other than the "semi-informative priors" method discussed previously. ↩
-
Of course, this isn't to say the estimates are completely independent of intuitions - intuitions are likely to color our choices of estimates for many of the difficult-to-estimate figures. But the ability to scrutinize and debate each estimate separately is helpful here. ↩